What is Contextual AI?
LILT uses Contextual AI models to provide translation suggestions. Contextual AI is a form of artificial intelligence that can understand and interpret the context of a given situation or query to provide fine tuned responses or outputs.
LILT has two types of models:
Baseline language models: The baseline model is shared across all customers. Each language pair has its own baseline model. LILT typically improves baseline models once a year using general-purpose data. Baseline models do not adapt based on new data.
Contextual AI models: For every Data Source, LILT creates a paired fine-tuned model on top of the baseline language model. This is specific to the Data Source, content context, and real-time adaptation through the human feedback cycle during translation in LILT, creating a specialized ability to generate on-brand, in-context translations.
Contextual AI is Interactive
Interactivity refers to the process of integrating AI suggestions into the translation workflow.
When translating in LILT, the Contextual AI intelligently suggests translations of the source segment that can be used during human translation.
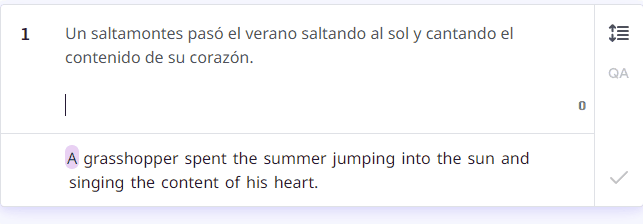
Contextual AI is Adaptive
'Adaptive' refers to the ability of LILT’s translation models to change in response to confirmed segments.
When a translator confirms a segment, the Contextual AI model processes the source/target pair as correct and uses it as an example to learn from when providing future translation suggestions. In essence, the translator teaches the AI system to adapt translation suggestions to the style, grammar, and word choice of the translator.
Adaptation speed differs across Data Sources. In some cases, adaptation will pick up on updated terminology in the very next segment. In other cases, it may take longer. The context of a segment is also important when referring to adaptation speed. For example, the translation of a particular segment may be used when the AI provides suggestions for nearby segments; however, the AI may fall back to its original suggestions for segments farther away in the document.